Mengjie Zhao
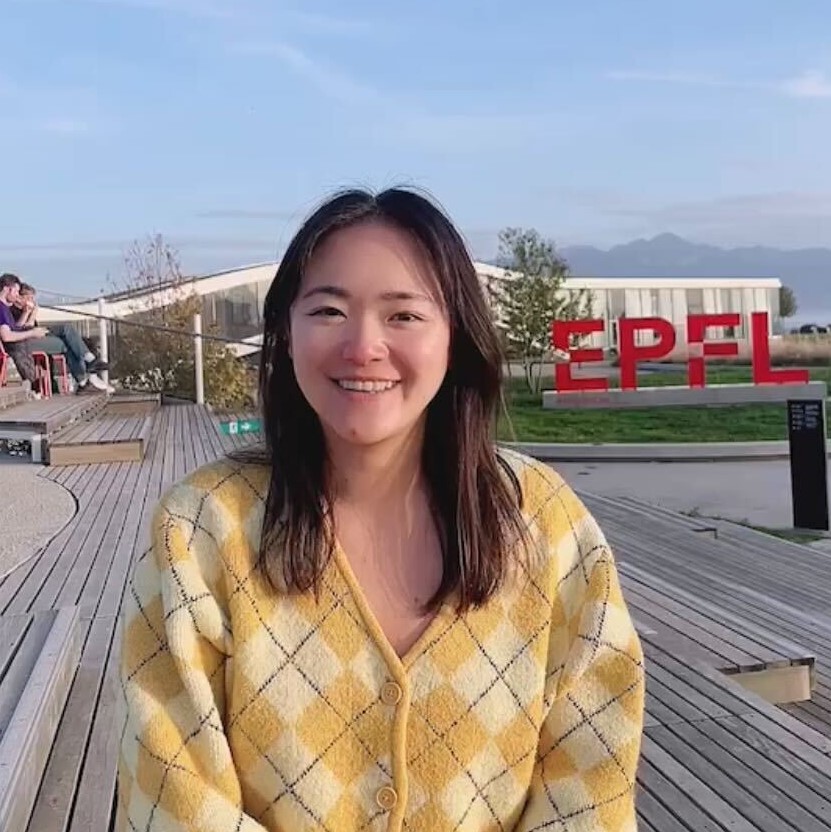
EPFL ENAC IIC IMOS
GC A3 434 (Bâtiment GC)
Station 18
1015 Lausanne
Web site: Web site: https://IMOS.epfl.ch
Biography
Mengjie Zhao holds degrees in Computational Mechanics (MSc) with honors track and in Engineering Science (BSc) from the Technical University of Munich (TUM). She has a background in numerical methods, specifically in turbulence modeling and computational fluid dynamics. Currently, she is working on learning the dynamics of complex systems with Graph Neural Networks (GNNs). In particular, she aims to improve the quality of predictive maintenance decisions by considering multiscale system dynamics and fusing heterogeneous sensory data.Education
M.Sc (with honors)
Computational Mechanics
Technical University of Munich
2017-2020
B.Sc
Engineering Science
Technical University of Munich
2014-2017
Research
Predictive Maintenance
In today's modern world, industrial and infrastructure systems are becoming increasingly complex and interdependent. These systems comprise many interconnected subsystems and components and operate under varying conditions that introduce non-linear and stochastic dynamics. To ensure their reliable and smooth operation, predictive maintenance (PM) is crucial. PM aims to predict when equipment is likely to fail and proactively address the issue before it causes significant damage. Effective PM can reduce downtime, increase system reliability, and save costs associated with emergency repairs or unavailability.Furthermore, complex systems are characterized not only by nonlinear dynamics, varying operating conditions, and hierarchical structure but also by a large number of heterogeneous multi-source system state data and rich interaction relations. These factors make it challenging to model and predict the behaviour of these systems effectively.
Temporal Graph Neural Networks
Despite the growing interest in using machine learning for PM, current deep learning algorithms are often not able to fully capture the complex, non-linear relationships present in these systems and lack robustness to varying operating conditions. Temporal Graph Neural Networks (TGNNs) offer a powerful solution for handling time series data with complex relationships. TGNNs can capture nonlinear, heterogenous, inter-component relationships under varying operating conditions by capturing both spatial and temporal structures of the graphs.The key challenges in TGNN-based PM are to effectively transform raw heterogeneous system data into a graph representation that captures the diverse, dynamic, and hierarchical interactions between time series under varying operating conditions, as well as designing appropriate network structures to model these interactions to extract system-level information for decision making.
My research aims to construct a TGNN-based framework capable of learning the dynamics of intricate industrial systems, thereby enhancing PM.