Federico Amato
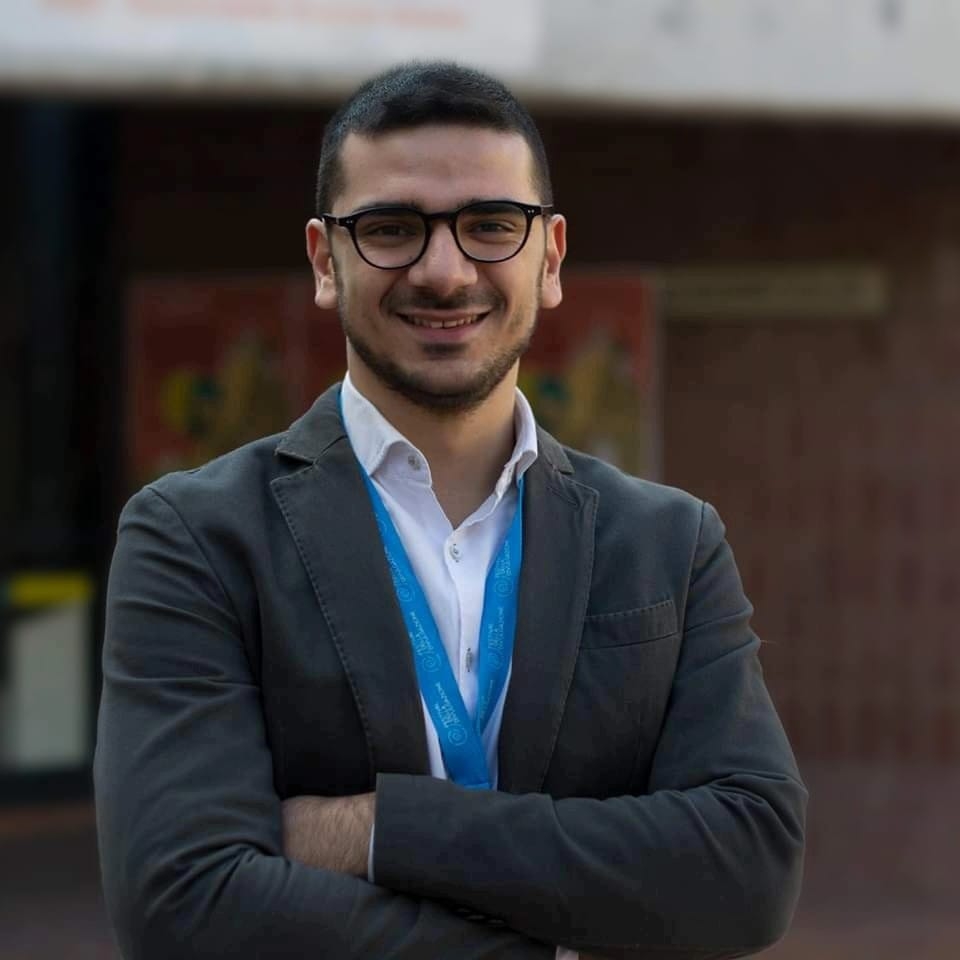
federico.amato@epfl.ch +41 21 693 12 36
Birth date: 12.08.1989
EPFL SDSC
INN 218 (Bâtiment INN)
Station 14
1015 Lausanne
+41 21 693 12 36
Office:
INN 218
EPFL
>
VPA-AVP-CP
>
SDSC
>
SDSC-GE
Web site: Web site: https://www.datascience.ch
Fields of expertise
Biography
I have an MSc in Engineering and a Ph.D. in Sustainable Development and Innovation Engineering. After a three-year Postdoc in Environmental Data Mining, in 2021 I joined the Swiss Data Science Centre, a joint venture between the polytechnical schools of Lausanne (EPFL) and Zurich (ETH) having as mission the acceleration of the digital transformation of the academic community and the industrial sector, putting to work Artificial Intelligence and Machine Learning and facilitating the multidisciplinary exchange of data and knowledge.Over the years I worked on the development of methodological tools to mine and model big spatiotemporal datasets. I extensively applied such methods to study the interaction between the spatial and temporal components of environmental and geographical phenomena, and their consequences in terms of spatial planning and urban geography. My academic activity has focused on the analysis of land-use dynamics and their relationship with climate, pollution, natural hazards, and other social/economic phenomena. I have deep competencies in applied statistics, machine learning, geocomputation, spatial statistics, urban modeling, and remote sensing. I am also an expert in European Project development and implementation.
Professional course
Postdoctoral Research Fellow
Environmental Data Mining
University of Lausanne
2018-2021
Education
Ph.D.
Innovation and Sustainable Development Engineering
University of Basilicata, Italy
2014-2018
Master’s degree
Engineering
University of Basilicata, Italy
2007-2014
Publications
Selected publications
Amato, F., Guignard, F., Robert, S., and Kanevski, M. Scientific reports, 10(1), 1-11. |
A novel framework for spatio-temporal prediction of environmental data using deep learning. |
Amato, F., Guignard, F., Walch, A., Mohajeri, N., Scartezzini, J. L., & Kanevski, M. arXiv preprint arXiv:2108.00859 |
Spatio-temporal estimation of wind speed and wind power using machine learning: predictions, uncertainty and technical potential. |
Amato, F., Guignard, F., Humphrey, V., & Kanevski, M. Association for Computing Machinery, Proceedings of the 10th International Conference on Climate Informatics, 37-43. |
Spatio-temporal evolution of global surface temperature distributions. |
Guignard, F., Amato, F., & Kanevski, M. Neurocomputing, 456, 436-449. |
Uncertainty quantification in extreme learning machine: Analytical developments, variance estimates and confidence intervals. |
Martellozzo, F., Amato, F., Murgante, B., & Clarke, K. C. Applied Geography, 91, 156-167. |
Modelling the impact of urban growth on agriculture and natural land in Italy to 2030. |